Very few know about the history of Artificial Intelligence. However, in recent decades, AI has become one of the market boom as well as the field of interest. AI is an old concept of the ’50s. But due to some complications and lack of government funding, the research collapsed. But IBM is the company that brought it to life once again in the ’90s. Since then, the AI is improving and the number of research is being performed, Furthermore, different companies are developing new hardware for the same purpose.
Artificial Intelligence – Field of interestÂ
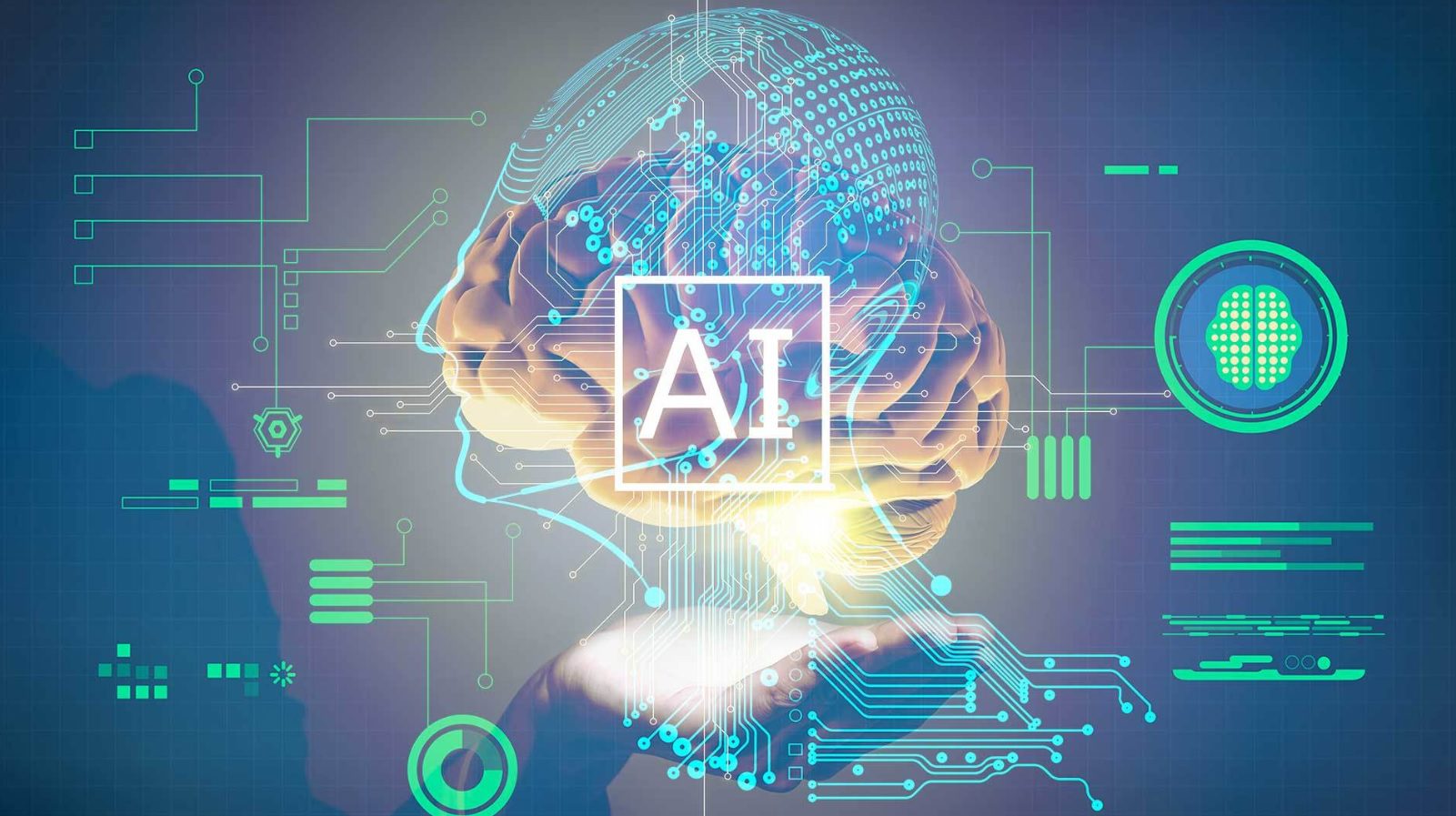
The brain researchers have learned a lot about the direct as well as indirect connections in the brain and about how the nervous system routes information and processes it. But there is still a huge amount of mystery yet unsolved.
Furthermore, computer algorithms, software, and hardware advances have brought Artificial Intelligence study to some other level. Besides, More and more universities are coming forward to provide ML, AI, and Deep Learning courses. Besides, the recent brain research and the ML are going in a parallel way. So we can say artificial intelligence and neuroscience-intercepting fields.
You must check What is The Difference Between Artificial Intelligence, Machine Learning, and Deep Learning.
A brain doesn’t work like a machine
People often label Artificial Intelligence as Machine Learning. Machine learning systems are better than human beings at finding complex patterns in a very large amount of data sets.
Self-driving cars, facial recognition software, financial fraud detection, robotics are using the same thing, ML. But to be honest, these all are just variations on a single statistical-based algorithm. Consequently, you will find a lot of similarities in different ML codes.
What’s the difference
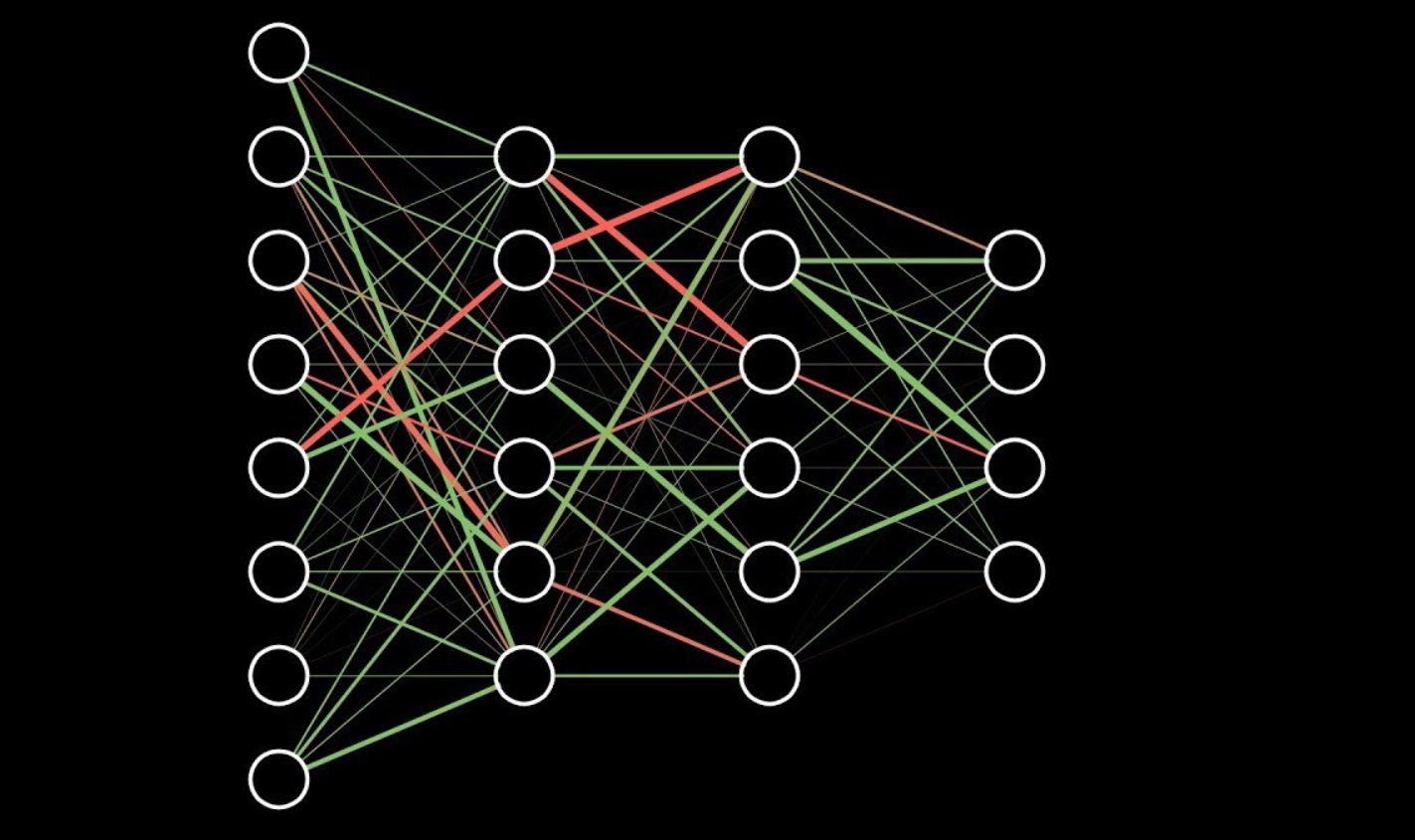
Neural networks are common to the mainstream approach to machine learning. These are highly interconnected networks of digital processors that accept inputs, then, process measurements about those inputs, and gives output. An ML model must learn what outputs should result from various inputs. The model learns from the training. Until the model develops the ability to respond to similar patterns in similar ways, the training goes on.
For instance, If you want a ML model to display the text “This is a cake” when it is shown a photo of a cow, you’ll have to give it a huge number of different photos of different types of cakes, photos from different angles so that it can adjust its neural network connections in order to recognize cake and give output, “This is a cake” to each one. Furthermore, if you show this model an image of a Biscuit, it will know only that it’s not a cake but can’t say what it is.
However, that’s not the way a brain learns, nor the way it handles the information to make sense of the surrounding. The brain takes in a very little amount of input data. For instance, a photograph of a cake and a drawing of a cow. After seeing only a very little number of examples, a brain will get the idea what a cake looks like. And then, it can identify a cake in a new image, from different angles and of different colors for sure.
A machine doesn’t work as a brain
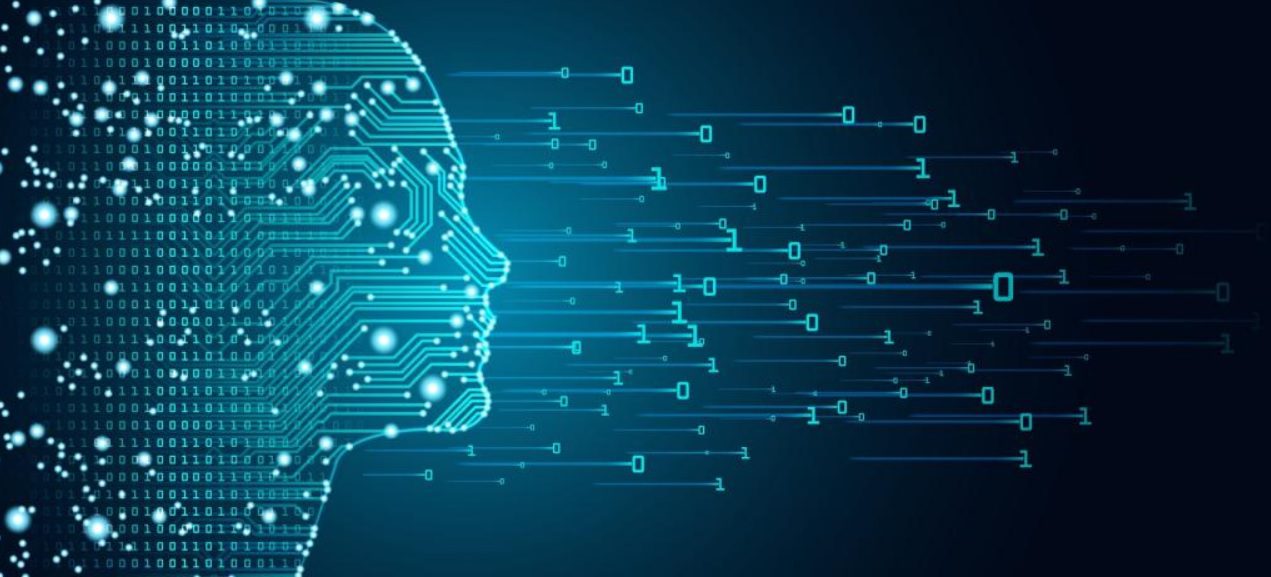
The brain can process information quite efficiently. Even when there’s noise or some distortion and uncertainty in input, the brain gets to give the correct output. Furthermore, under unpredictably changing conditions too, the brain works.
For instance, if you see an image of cake which is covered by something and only a little portion is visible, even then the brain can understand that its a cake. And in case the photo is distorted in terms of color, the brain will still get it, that its a cake. but an ML model sometimes can’t understand that. A distorted image of a ripped image or partially covered image will reduce the accuracy of the model.
The brain can also work with some injury, but think of, will a half-burnt circuit work? The hardware has to be perfectly fine. Besides, there is also a matter of power. A lot of time, as well as power, is needed to train an Artificial Intelligence model. On the other hand, a brain takes a very little amount of time as well as the power to recognize some features. So, we can say the brain is more efficient than the ML model.
On the other hand, it’s also true that a brain cant analyzes a big amount of data. For instance, a brain can’t take the weather data of 10 or 20 years and predict the weather of a particular day. but an ML model can certainly take that amount of data and can predict the weather of a particular day.
Combining Neuroscience and Artificial Intelligence
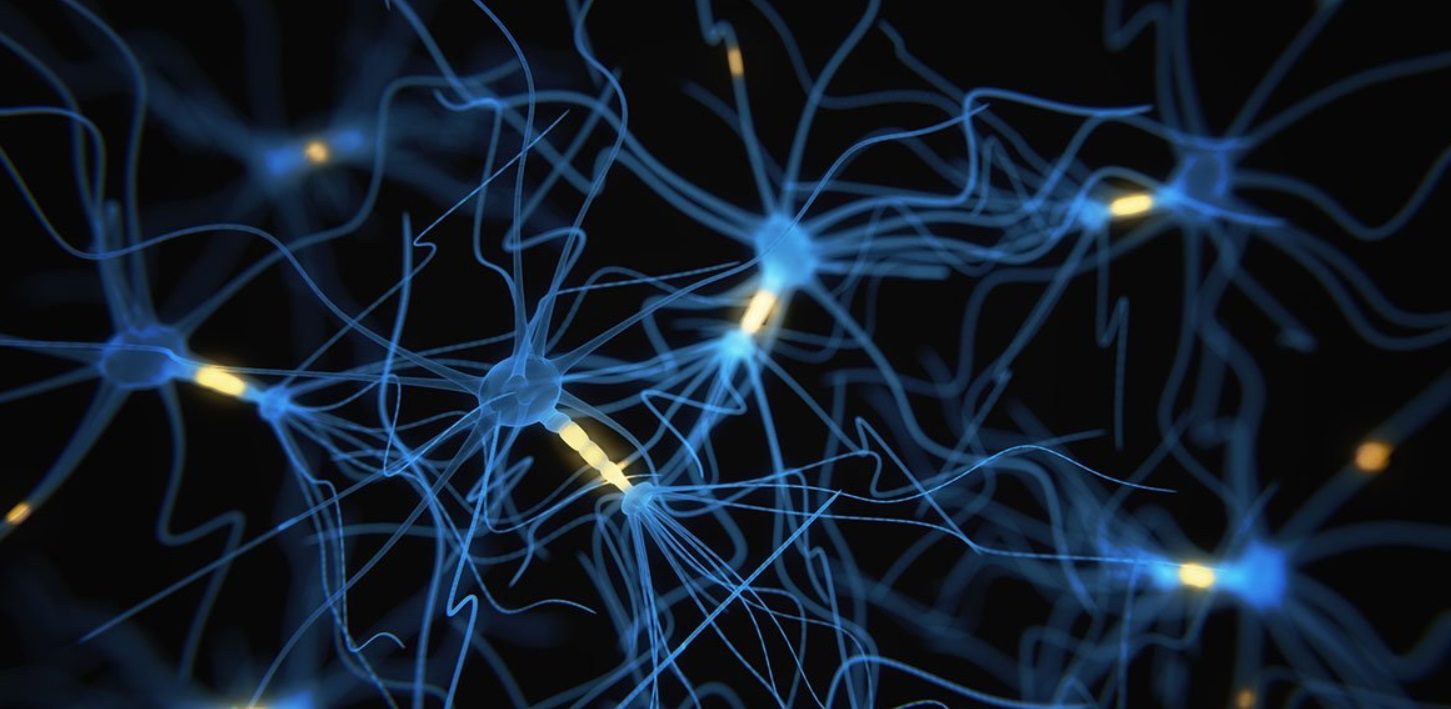
Indeed artificial intelligence and neuroscience-intercepting fields. However, discovering how the brain works, don’t clear which brain processes might work well as machine learning algorithms. the only way to understand the function of the brain a neuroscientist may help. On the other hand, an Artificial Intelligence researcher can understand the function to improve the ML model efficiency.
For instance, we can connect the working concept of the brain’s neurons to the neurons of the ML model. As far we know, the Individual neuron of the ML model, communicates to the other individual neurons irrespective of the output and the input. No matter how big the network is, the local interactions collectively influence the activity of the whole network.
The mathematics that describes these layers of artificial neural networks are also applicable in biological neural networks in real brains. Consequently, researchers are developing a new form of machine learning that can learn without training. Certainly, that will be efficient.
Besides, Researchers have used those ideas to explore why the brain’s neurons have twisted and convoluted shapes. So, they found that the shape makes the recognization as well as the training more efficient. Because the numbers of interconnections are more.
Take away
To conclude, we can say, the Artificial Intelligence and the neuroscience are merging to open up new possibilities of the ML. Consequently, we can say, artificial intelligence and neuroscience-intercepting fields.